
Prof. Shaohua Wan
University of Electronic Science and Technology of China, China
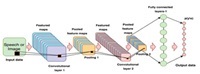
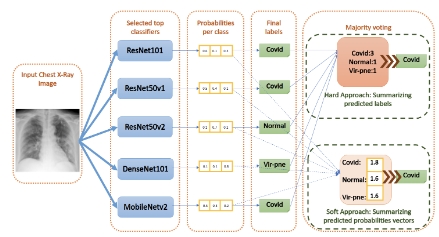
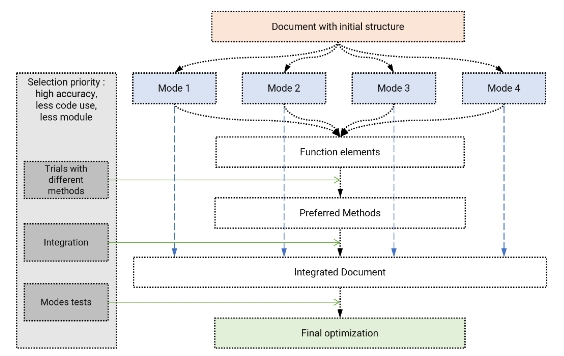
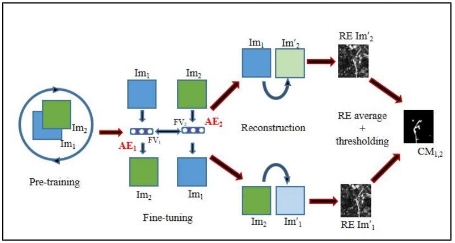
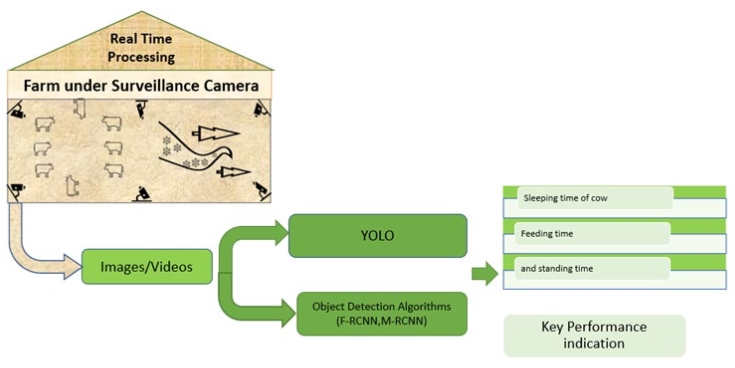
Open Access
Article
Article ID: 1450
by Bushra Sajid, Ahmed Abu-Halimeh, Nuh Jakoet
Computing and Artificial Intelligence, Vol.3, No.1, 2025; 107 Views, 62 PDF Downloads
Entity Resolution (ER) has been investigated for decades in various domains as a fundamental task in data integration and data quality. The emerging volume of heterogeneously structured data and even unstructured data challenges traditional ER methods. This research mainly focuses on the Data Washing Machine (DWM). The DWM was developed in the NSF DART Data Life Cycle and Curation research theme, which helps to detect and correct certain types of data quality errors automatically. It also performs unsupervised entity resolution to identify duplicate records. However, it uses traditional methods that are driven by algorithmic pattern rules such as Levenshtein Edit Distances and Matrix comparators. The goal of this research is to assess the replacement of rule-based methods with machine learning and deep learning methods to improve the effectiveness of the processes using 18 sample datasets. The DWM has different processes to improve data quality, and we are currently focusing on working with the scoring and linking processes. To integrate the machine model into the DWM, different pre-trained models were tested to find the one that helps to produce accurate vectors that can be used to calculate the similarity between the records. After trying different pre-trained models, distilroberta was chosen to get the embeddings, and cosine similarity metrics were later used to get the similarity scores, which helped us assess the machine learning model into DWM and gave us closer results to what the scoring matrix is giving. The model performed well and gave closer results overall, and the reason can be that it helped to pick up the important features and helped at the entity matching process.
Open Access
Article
Article ID: 1498
by Zarif Bin Akhtar
Computing and Artificial Intelligence, Vol.3, No.1, 2025; 211 Views, 133 PDF Downloads, 3 Supp. file Downloads
This research explores the transformative integration of artificial intelligence (AI), robotics, and language models, with a particular emphasis on the PaLM-E model. The exploration aims to assess PaLM-E’s decision-making processes and adaptability across various robotic environments, demonstrating its capacity to convert textual prompts into very precise robotic actions. In addition, the research investigates Parameter-Efficient Fine-Tuning (PEFT) techniques, such as Low-Rank Adaptation (LoRA) and Quantized Low-Rank Adaptation (QLoRA), providing a historical overview of PEFT and highlighting their significance in enhancing task performance while reducing the number of trainable parameters. The broader scope of Generative AI is examined through an analysis of influential models like GPT-3, GPT-4, Copilot, Bard, LLaMA, Stable Diffusion, Midjourney, and DALL-E. These models’ abilities to process natural language prompts and generate a wide range of outputs are thoroughly investigated. The research traces the historical evolution of AI, from its roots in science fiction to its practical applications today, with a focus on the rise of Generative AI in the 21st century. Furthermore, the research delves into the various modalities of Generative AI, covering applications in text, code, images, and more, and assesses their real-world impact on robotics, planning, and business intelligence. The implications of synthetic data generation for business analytics are also explored. The research inspects within both software and hardware landscapes, comparing local deployment on consumer-grade hardware along with cloud-based services, and underscores the benefits of local model deployment in terms of privacy protection, intellectual property security, and censorship resistance. Ethical considerations are central to this research, addressing concerns related to privacy, security, societal impact, biases, and misinformation. The research proposes ethical guidelines for the responsible development and deployment of AI technologies. Ultimately, this work reveals the deep interconnections between vision, language, and robotics, pushing the boundaries of AI capabilities and providing crucial insights for future AI model development and technological innovation. These findings are intended to guide the field through the emerging challenges of the rapidly evolving Generative AI landscape.
Open Access
Article
Article ID: 1489
by Safdar Hayat, Rahila Anwar, Sartaj Aziz
Computing and Artificial Intelligence, Vol.3, No.1, 2025; 32 Views, 15 PDF Downloads
In this research, an advanced artificial neural network (ANN)-based approach for prognosis and classification of dengue disease is presented. Dengue diagnosis usually relies on clinical assessment; subsequently, there might be a high probability of misdiagnoses due to the complex hodgepodge of symptoms of dengue with other vector-borne diseases. It is needed to develop a system that can help doctors to identify dengue disease much faster than the manual system, which takes longer time and more cost to detect the diseases. Such a system may help users to take an early action before it becomes serious. The study involved three phases: pre-processing, neural network processing, and post-processing. In the pre-processing phase, data were gathered from three high-severity dengue outbreak sites in Pakistan (Benazir Bhutto Hospital, CITI Lab Rawalpindi, and Meo Hospital Lahore) where the dengue outbreak severity was high during the year of 2011. After cleaning and normalizing, 768 samples were obtained, split into 560 for training and 208 for testing. Nineteen critical parameters were selected with input from physicians, medical staff, and prior research. This study presents a supervised feed-forward neural network (FFNN) with two hidden layers, trained using backpropagation and optimized with the Levenberg-Marquardt algorithm, achieving nearly 100% accuracy, minimal runtime, and a very low MSE (0.00000000000032521). The model reached 100% sensitivity, 99.8% precision, and 98.7% specificity, surpassing prior results in dengue diagnosis. The findings support improved diagnostic accuracy and confidence, providing a framework for physicians. Key factors in achieving optimal results include careful selection of architecture, data normalization, parameter selection, and critical evaluation.
Open Access
Article
Article ID: 1934
by Surajit Mondal, Shankha Shubhra Goswami
Computing and Artificial Intelligence, Vol.3, No.1, 2025; 31 Views, 14 PDF Downloads
This paper focuses on assessing the Economic Impact (EI) of Cloud Computing (CC), which has emerged as a powerful technology that can transform business operations and enhance economic growth. This paper employs a narrative literature review methodology to assess the EI of CC, which has emerged as a transformative technology. It begins by examining the economic benefits of CC, including cost savings, improved efficiency, and increased innovation. Subsequently, it explores the challenges associated with assessing the EI of CC, such as data privacy and security concerns, interoperability issues, and the need for new regulatory frameworks. The paper also provides insights into the opportunities and challenges that CC presents for different sectors of the economy, including healthcare, finance, and government. Ultimately, the paper emphasizes the importance of a holistic approach to assessing the EI of CC that considers both its benefits and challenges in order to make informed decisions about its adoption and use.
Open Access
Article
Article ID: 1987
by Erdem Çetin, Murat Berker Özbek, Sezin Biner, Ceren Ulus, M. Fatih Akay
Computing and Artificial Intelligence, Vol.3, No.1, 2025; 42 Views, 19 PDF Downloads
In the clothing sector, matching the right demand with the appropriate user is of great significance. Combination suggestions emerge as an innovative strategy for e-commerce platforms operating in the clothing sector. By providing suitable combination suggestions tailored to the right user, the profit margin of sales increased, and the brand image strengthened. The aim of this study is to develop a recommendation system based on image processing and machine learning that generates combinations from products that may interest users and recommends these combinations to them. 90 million possible combinations have been obtained using a dataset consisting of products detected from images of items sold in the clothing category on Trendyol. These combinations have been trained using the Prod2Vec algorithm to create new pairings. Subsequently, collections have been developed for purchasing looks using image processing methods. In this context, the You Only Look Once (YOLO) model has been selected for clothing classification, while the Convolutional Network Next (ConvNext) model has been employed for calculating image similarity. Models have also been developed for estimating click performance using Random Forest (RF), Extreme Gradient Boosting (XGBoost), and Linear Regression (LR). The prediction performances of the developed models have been evaluated using Coefficient of Determination ( R 2 ), Mean Squared Error (MSE), and Mean Absolute Error (MAE) metrics. When the developed models have been examined, it has been observed that RF had superior performance. The developed system provided a 5% increase in the time spent on the Trendyol mobile application.
Open Access
Article
Article ID: 2018
by Terecio Diosnel Marecos Brizuela, David Luis La Red Martínez, Federico Agostini, Jorge Tomás Fornerón Martínez
Computing and Artificial Intelligence, Vol.3, No.1, 2025; 22 Views, 15 PDF Downloads
In distributed processing environments, multiple groups of processes are found sharing resources and competing for access. These processes may or may not require synchronization and it is essential to reach a consensus to manage access to resources in a way that establishes a strict order, thus ensuring mutual exclusion. The proposal presented is an innovative and effective solution for the management of shared resources in distributed systems, which allows solving problems related to overload and workload balancing. The evaluation of the state of computational loads and the final comparison using standard deviation are useful tools to detect and correct imbalances in the system. In addition, the possibility of establishing initial configurations of the algorithm for each particular situation allows adapting the solution to the specific needs of each system.
Open Access
Article
Article ID: 1577
by P. Saranya, R. Durga
Computing and Artificial Intelligence, Vol.3, No.1, 2025; 13 Views, 10 PDF Downloads
Segmentation of nut images plays a vital role in computer vision and agricultural applications. Precise segmentation enables the extraction and analysis of essential information about the nuts, supporting quality evaluation, yield estimation, and automated sorting processes. This study explores nuts image segmentation utilizing the cuckoo search algorithm. The cuckoo search algorithm, a nature-inspired optimization technique, is introduced to enhance the segmentation process, potentially optimizing parameters or guiding the segmentation algorithms. Performance evaluation emphasizes metrics such as MSE, IoU, and dice coefficient. CSA (cuckoo search algorithm) demonstrates superior results, showcasing its effectiveness in automated nuts segmentation. This research contributes to the advancement of nut image analysis, providing insights into segmentation methodologies that can enhance automated processes in agriculture and food industry applications. The findings underscore the significance of employing advanced algorithms like CSA for accurate and efficient segmentation of nuts in images.
Open Access
Perspective
Article ID: 1581
by Madhab Chandra Jena, Sarat Kumar Mishra, Himanshu Sekhar Moharana
Computing and Artificial Intelligence, Vol.3, No.1, 2025; 80 Views, 37 PDF Downloads
Artificial Intelligence (AI) is revolutionizing various sectors, including healthcare, finance, and education, yet its rapid adoption is accompanied by significant challenges and drawbacks that warrant urgent attention. This manuscript explores key issues such as job displacement, algorithmic bias, privacy concerns, and environmental impacts, presenting a comprehensive overview of the multifaceted challenges associated with AI integration. Utilizing a robust methodology that includes literature reviews, thematic analysis, and expert interviews, the study identifies critical barriers to effective AI implementation. Furthermore, it proposes strategic recommendations aimed at mitigating these challenges, emphasizing the need for reskilling initiatives, ethical frameworks, and collaborative regulatory efforts. The findings underscore the importance of a balanced approach that maximizes AI benefits while addressing its inherent risks, ultimately paving the way for a more equitable and sustainable technological future.