
Prof. Shaohua Wan
University of Electronic Science and Technology of China, China
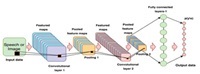
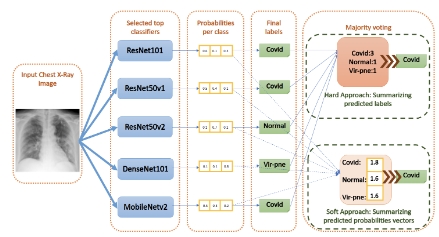
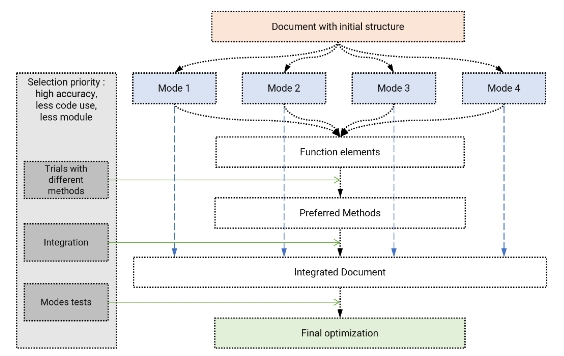
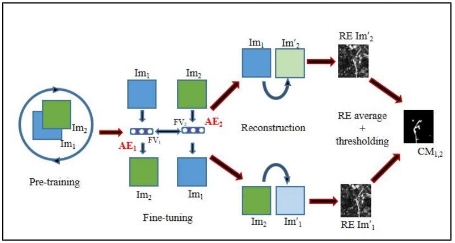
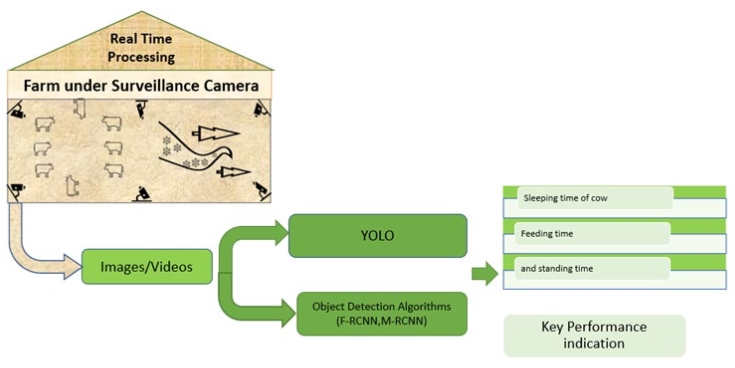
Open Access
Article
Article ID: 545
by Hmidi Alaeddine, Malek Jihene
Computing and Artificial Intelligence, Vol.2, No.2, 2024; 335 Views, 191 PDF Downloads
In this work, an effort is made to apply the FractalNet model in the field of plant disease classification. The proposed model was trained and tested using a “PlantVillage” plant disease image dataset using a central processing unit (CPU) environment for 300 epochs. It produced an average classification accuracy of 99.9632% on the test dataset. The experimental results demonstrate the efficiency of the proposed model and show that the model achieved the highest values compared to other deep learning models in the PlantVillage datasets.
Open Access
Article
Article ID: 1409
by Mohan Bhandari, Sushant Shrestha, Utsab Karki, Santosh Adhikari, Rajan Gaihre
Computing and Artificial Intelligence, Vol.2, No.2, 2024; 426 Views, 173 PDF Downloads
Deepfake technology, which uses artificial intelligence to create and manipulate realistic synthetic media, poses a serious threat to the trustworthiness and integrity of digital content. Deepfakes can be used to generate, swap, or modify faces in videos, altering the appearance, identity, or expression of individuals. This study presents an approach for deepfake detection, based on a convolutional vision transformer (CViT), a hybrid model that combines convolutional neural networks (CNNs) and vision transformers (ViTs). The proposed study uses a 20-layer CNN to extract learnable features from face images, and a ViT to classify them into real or fake categories. The study also employs MTCNN, a multi-task cascaded network, to detect and align faces in videos, improving the accuracy and efficiency of the face extraction process. The method is assessed using the FaceForensics++ dataset, which comprises 15,800 images sourced from 1600 videos. With an 80:10:10 split ratio, the experimental results show that the proposed method achieves an accuracy of 92.5% and an AUC of 0.91. We use Gradient-Weighted Class Activation Mapping (Grad-CAM) visualization that highlights distinctive image regions used for making a decision. The proposed method demonstrates a high capability of detecting and distinguishing between genuine and manipulated videos, contributing to the enhancement of media authenticity and security.
Open Access
Article
Article ID: 1364
by Ajay Jaiswal, Piyush Malviya, Lucky Parihar, Rani Pathak, Kuldeep Rajput
Computing and Artificial Intelligence, Vol.2, No.2, 2024; 218 Views, 107 PDF Downloads
This paper presents the design and implementation of a software cost estimation tool integrated into a mobile application developed using Flutter. The tool incorporates various techniques for software cost estimation, including expert judgment, function point analysis, 3D point analysis, and the COCOMO model. The purpose of the program is to give software engineers and project managers a practical and effective tool for calculating the time and money needed for software development projects. The paper provides a thorough explanation of each estimation technique’s implementation, along with a discussion of the app’s main features and functionalities. Because of the app’s intuitive and user-friendly design, users can quickly enter project data and get precise cost estimates. The tool’s efficacy is assessed using case studies and contrasts with other software cost estimation methods currently in use. The outcomes show that the app can produce trustworthy and precise cost estimates, which makes it an important resource for software development projects.
Open Access
Article
Article ID: 1467
by Yuanyuan Xu, Weiting Gao, Yining Wang, Xinyang Shan , Yin-Shan Lin
Computing and Artificial Intelligence, Vol.2, No.2, 2024; 257 Views, 99 PDF Downloads
This study explores the enhancement of user experience (UX) and trust in advanced Large Language Model (LLM)-based conversational agents such as ChatGPT. The research involves a controlled experiment comparing participants using an LLM interface with those using a traditional messaging app with a human consultant. The results indicate that LLM-based agents offer higher satisfaction and lower cognitive load, demonstrating the potential for LLMs to revolutionize various applications from customer service to healthcare consultancy and shopping assistance. Despite these positive findings, the study also highlights significant concerns regarding transparency and data security. Participants expressed a need for clearer understanding of how LLMs process information and make decisions. The perceived opacity of these processes can hinder user trust, especially in sensitive applications such as healthcare. Additionally, robust data protection measures are crucial to ensure user privacy and foster trust in these systems. To address these issues, future research and development should focus on enhancing the transparency of LLM operations and strengthening data security protocols. Providing users with clear explanations of how their data is used and how decisions are made can build greater trust. Moreover, specialized applications may require tailored solutions to meet specific user expectations and regulatory requirements. In conclusion, while LLM-based conversational agents have demonstrated substantial advantages in improving user experience, addressing transparency and security concerns is essential for their broader acceptance and effective deployment. By focusing on these areas, developers can create more trustworthy and user-friendly AI systems, paving the way for their integration into diverse fields and everyday use.
Open Access
Article
Article ID: 1447
by Erin Chelsea Hathorn, Ahmed Abu Halimeh
Computing and Artificial Intelligence, Vol.2, No.2, 2024; 94 Views, 47 PDF Downloads
In the ever-expanding landscape of digital technologies, the exponential growth of data in information science and health informatics presents both challenges and opportunities, demanding innovative approaches to data curation. This study focuses on evaluating various feasible clustering methods within the Data Washing Machine (DWM), a novel tool designed to streamline unsupervised data curation processes. The DWM integrates Shannon Entropy into its clustering process, allowing for adaptive refinement of clustering strategies based on entropy levels observed within data clusters. Rigorous testing of the DWM prototype on various annotated test samples revealed promising outcomes, particularly in scenarios with high-quality data. However, challenges arose when dealing with poor data quality, emphasizing the importance of data quality assessment and improvement for successful data curation. To enhance the DWM’s clustering capabilities, this study explored alternative unsupervised clustering methods, including spectral clustering, autoencoders, and density-based clustering like DBSCAN. The integration of these alternative methods aimed to augment the DWM’s ability to handle diverse data scenarios effectively. The findings demonstrated the practicability of constructing an unsupervised entity resolution engine with the DWM, highlighting the critical role of Shannon Entropy in enhancing unsupervised clustering methods for effective data curation. This study underscores the necessity of innovative clustering strategies and robust data quality assessments in navigating the complexities of modern data landscapes. This content is structured by the following sections: Introduction, Methodology, Results, Discussion, and Conclusion.
Open Access
Article
Article ID: 1443
by Yongquan Yang, Hong Bu
Computing and Artificial Intelligence, Vol.2, No.2, 2024; 97 Views, 23 PDF Downloads, 2 Supplementary materials Downloads
The logical assessment formula (LAF) is a new theory proposed for evaluations with inaccurate ground-truth labels (IAGTLs) to assess the predictive models for artificial intelligence applications. However, the practicability of LAF for evaluations with IAGTLs has not yet been validated in real-world practice. In this paper, we applied LAF to two tasks of tumour segmentation for breast cancer (TSfBC) in medical histopathology whole slide image analysis (MHWSIA) for evaluations with IAGTLs. Experimental results and analysis show that the LAF-based evaluations with IAGTLs were unable to confidently act like usual evaluations with accurate ground-truth labels on the one easier task of TSfBC while being able to reasonably act like usual evaluations with AGTLs on the other more difficult task of TSfBC. These results and analysis reflect the potential of LAF applied to MHWSIA for evaluations with IAGTLs. This paper presents the first practical validation of LAF for evaluations with IAGTLs in a real-world application.
Open Access
Article
Article ID: 1291
by Claudio Zancan, João Luiz Passador, Cláudia Souza Passador, Ricardo Carvalho Rodrigues
Computing and Artificial Intelligence, Vol.2, No.2, 2024; 111 Views, 75 PDF Downloads
This study aims to address the existing knowledge gap regarding the specific impact of artificial intelligence (AI) on patent research and emphasize its strategic significance as a catalyst for innovation. The methodology employs a comprehensive approach, integrating both qualitative and quantitative research methods. It systematically investigates the transformative potential of AI in patent research within the BRICS nations, including an examination of the technological, ethical, and legal challenges associated with AI’s application in patent analysis. This research contributes to the field by extending beyond the conventional focus on the role of patents in innovation and shedding light on the potential of AI in patent research. It offers valuable insights into how AI can redefine the landscape of patent research, providing a more rapid and accurate perspective on the identification of technological trends, opportunities, and competitive factors. The findings underscore that AI in patent research yields numerous advantages, ranging from efficient data processing to the forecasting of technological trends. Future studies should explore ethical and legal considerations associated with AI in patent research, as well as its implementation in the strategies of both corporate entities and governmental bodies in the BRICS nations.
Open Access
Article
Article ID: 570
by C. Rajabhushanam
Computing and Artificial Intelligence, Vol.2, No.2, 2024; 179 Views, 84 PDF Downloads
In this study, the author proposes and details a workflow for the spatial-temporal demarcation of urban areal features in 8 cities of Tamilnadu, India. During the inception phase, functional requirements and non-functional parameters are analyzed and designed, within a suitable pixel area and object-oriented derived paradigm. Land use categories are defined from OpenStreetMap (OSM) related works with the scope of conducting climate change, using multispectral sensors onboard Landsat series. Furthermore, we augment the bands dataset with Spatially Invariant Feature Transform (SIFT), Normalized Difference Vegetation Index (NDVI), Normalized Difference Built-Up Index (NDBI), Leaf Area Index (LAI), and Texture base indices, as a means of spatially integrating auto-covariance to stationarity patterns. In doing so, change detection can be pursuit by scaling up the segmentation of regional/zonal boundaries in a multi-dimensional environment, with the aid of Wide Area Networks (WAN) cluster computers such as the BEOWULF/Google Earth Engine clusters. GeoAnalytical measures are analyzed in the design of local and zonal spatial models (GRID, RASTER, DEM, IMAGE COLLECTION). Finally, multi variate geostatistical works are ensued for precision and recall in predictive data analytics. The author proposes reusing machine learning tools (filtering by attribute-based indexing in PaaS clouds) for pattern recognition and visualization of features and feature collection.
Open Access
Article
Article ID: 1481
by Sara Pouriamehr, Valiollah Dabidi Roshan, Somayeh Namdar Tajari
Computing and Artificial Intelligence, Vol.2, No.2, 2024; 51 Views, 16 PDF Downloads
Although the use of biofeedback devices is beyond measure, they are widely applied only for clinical purposes. Therefore, this study evaluated whether biofeedback devices could be applied to estimate heart rate variability (HRV) among healthy populations. 60 individuals (46 ± 5 years; 30 women) performed maximal exercise protocol (MEP). At pre- and post-MEP status, HRV indexes were collected by two devices: 1) the electrocardiogram device (ECG); 2) the biofeedback device (BIO). At pre-exercise status, all HRV parameters had significant correlations, ranging from low ( r = 0.241) to high ( r = 0.779). At post-exercise status, significant correlations for some of the HRV measures were found as well, ranging from low (i.e., r ≤ 0.29) to moderate (i.e., 0.3 ≤ r ≤ 0.49). According to our knowledge, this study is the first attempt to evaluate HRV by biofeedback devices among healthy individuals, which shows they can also be applied as a swift method to examine HRV among healthy individuals, especially in rest conditions.
Open Access
Article
Article ID: 1485
by Zarif Bin Akhtar, Ahmed Tajbiul Rawol
Computing and Artificial Intelligence, Vol.2, No.2, 2024; 109 Views, 31 PDF Downloads
The integration of artificial intelligence (AI) into cybersecurity has brought about transformative advancements in threat detection and mitigation, yet it also introduces new vulnerabilities and potential threats. This research exploration systematically investigates the critical issues surrounding AI within cybersecurity, focusing on specific vulnerabilities and the potential for AI systems to be exploited by malicious actors. The research aims to address these challenges by swotting and analyzing existing methodologies designed to mitigate such risks. Through a detailed exploration of modern scientific research, this manuscript identifies the dual-edged impact of AI on cybersecurity, emphasizing both the opportunities and the dangers. The findings highlight the need for strategic solutions that not only enhance digital security and user privacy but also address the ethical and regulatory aspects of AI in cybersecurity. Key contributions include a comprehensive analysis of emerging trends, challenges, and the development of AI-driven cybersecurity frameworks. The research also provides actionable recommendations for the future development of robust, reliable, and secure AI-based systems, bridging current knowledge gaps and offering valuable insights for academia and industry alike.
Open Access
Review
Article ID: 1279
by Yasunari Matsuzaka, Ryu Yashiro
Computing and Artificial Intelligence, Vol.2, No.2, 2024; 149 Views, 71 PDF Downloads
Similarly, to all coronaviruses, SARS-CoV-2 uses the S glycoprotein to enter host cells, which contains two functional domains: S1 and S2 receptor binding domain (RBD). Angiotensin-converting enzyme 2 (ACE2) is recognizable by the S proteins on the surface of the SARS-CoV-2 virus. The SARS-CoV-2 virus causes SARS, but some mutations in the RBD of the S protein markedly enhance their binding affinity to ACE2. Searching for new compounds in COVID-19 is an important initial step in drug discovery and materials design. Still, the problem is that this search requires trial-and-error experiments, which are costly and time-consuming. In the automatic molecular design method based on deep reinforcement learning, it is possible to design molecules with optimized physical properties by combining a newly devised coarse-grained representation of molecules with deep reinforcement learning. Also, structured-based virtual screening uses protein 3D structure information to evaluate the binding affinity between proteins and compounds based on physicochemical interactions such as van der Waals forces, Coulomb forces, and hydrogen bonds, and select drug candidate compounds. In addition, AlphaFold can predict 3D protein structures, given the amino acid sequence, and the protein building blocks. Ensemble docking, in which multiple protein structures are generated using the molecular dynamics method and docking calculations are performed for each, is often performed independently of docking calculations. In the future, the AlphaFold algorithm can be used to predict various protein structures related to COVID-19.