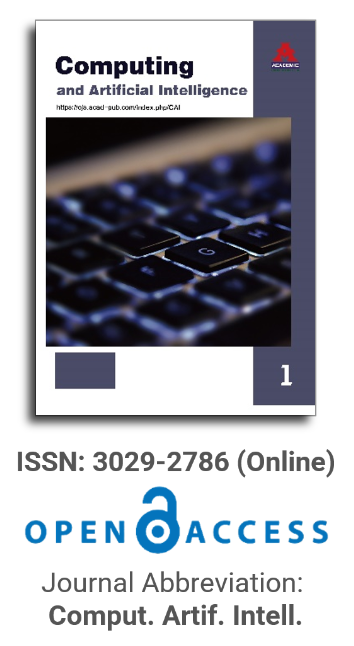
Description
Computing and Artificial Intelligence (CAI) is a peer-reviewed, open-access journal dedicated to the dissemination of cutting-edge research in the fields of computer science and artificial intelligence. The journal aims to bridge the gap between theoretical research and practical applications by providing a platform for scholars, researchers, and industry professionals to share their insights and findings. CAI is published bi-annual, ensuring a regular flow of new research findings and discussions. All the papers published in CAI could be accessed, read, and downloaded freely with the aims that making research freely available to the public, fostering greater collaboration and knowledge exchange within the scientific community.
The journal welcomes submissions from worldwide researchers, and practitioners in the field of Artificial Intelligence, which can be original research articles, review articles, editorials, case reports, commentaries, etc. Authors are encouraged to adhere to the submission guidelines provided on the journal's website to ensure a smooth review process.