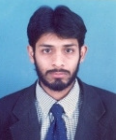
Assoc. Prof. Ahmad Zeeshan
International Islamic University, Pakistan
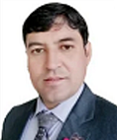
Dr. Nehad Ali Shah
Sejong University, Korea
Academic Publishing insists on taking academic exchange and publication as the main line, carrying out comprehensive management based on science and technology, and fully exploring excellent international publishing resources. Within 5 years, it will form a strategic framework and scale with science (S), technology (T), medicine (M), education (E), and humanities and arts (H) as the main publishing fields. Academic Publishing is headquartered in Singapore and based in Malaysia, with the United States and China providing the main scientific and academic resources. At the same time, it has established long-term good cooperative relations with other publishing companies, scientific research communities, and academic organizations in more than a dozen countries and regions. Academic Publishing uses English and Chinese as its main publishing languages, mainly publishing books, journals, and conference papers in print and online. The vast majority of publications follow the international open access policy, providing stable and long-term quality and professional publications. With the joint efforts of the expert team and our professional editorial team, our publications will gradually be indexed by international databases in stages to provide convenient and professional retrieval for various scholars. At the same time, manuscripts we accept will be subject to the peer review principle, and cutting-edge and innovative research articles will be preferentially accepted for peer reference and discussion. All kinds of our publications are welcome for peer to contribute, access, and download.
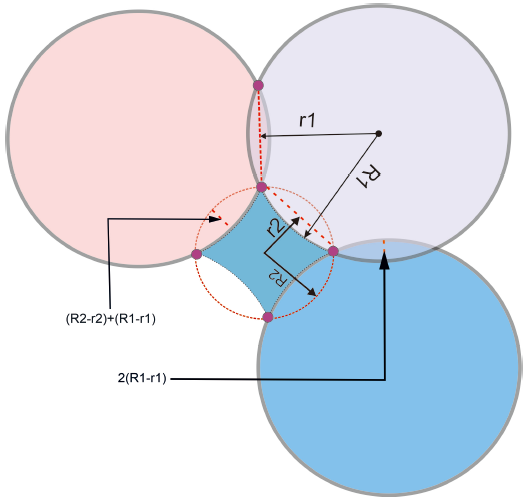
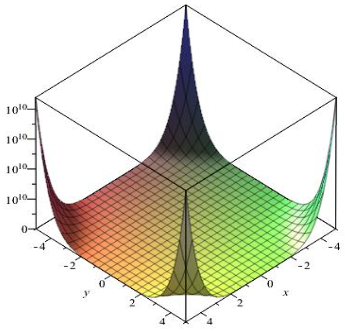
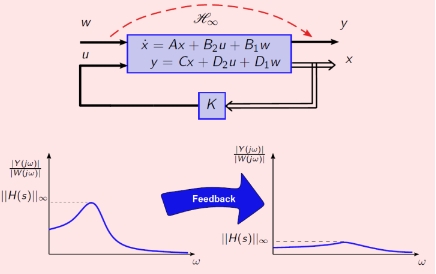
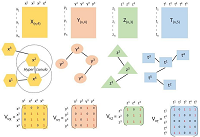
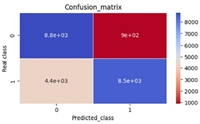